Model to help PWSA more efficiently find and replace lead service lines
Pittsburgh, PA -Over the past year, the Pittsburgh Water and Sewer Authority (PWSA) and the University of Pittsburgh’s School of Computing and Information (SCI) and University Center for Social and Urban Research (UCSUR) have collaborated on a machine learning model that will help better predict the locations of remaining lead service lines in PWSA’s water distribution system.
Since PWSA’s compliance testing exceeded the Environmental Protection Agency’s (EPA) action level for lead in 2016, we have been hard at work to identify the location of lead service lines, replace them with a non-lead material, and optimize the water treatment process to reduce the risk of lead at the approximately 8,000 homes with lead service lines. Through this partnership, PWSA will better be able to predict the locations of lead lines where no reliable record is found, avoiding costly excavations and impact to our customers.
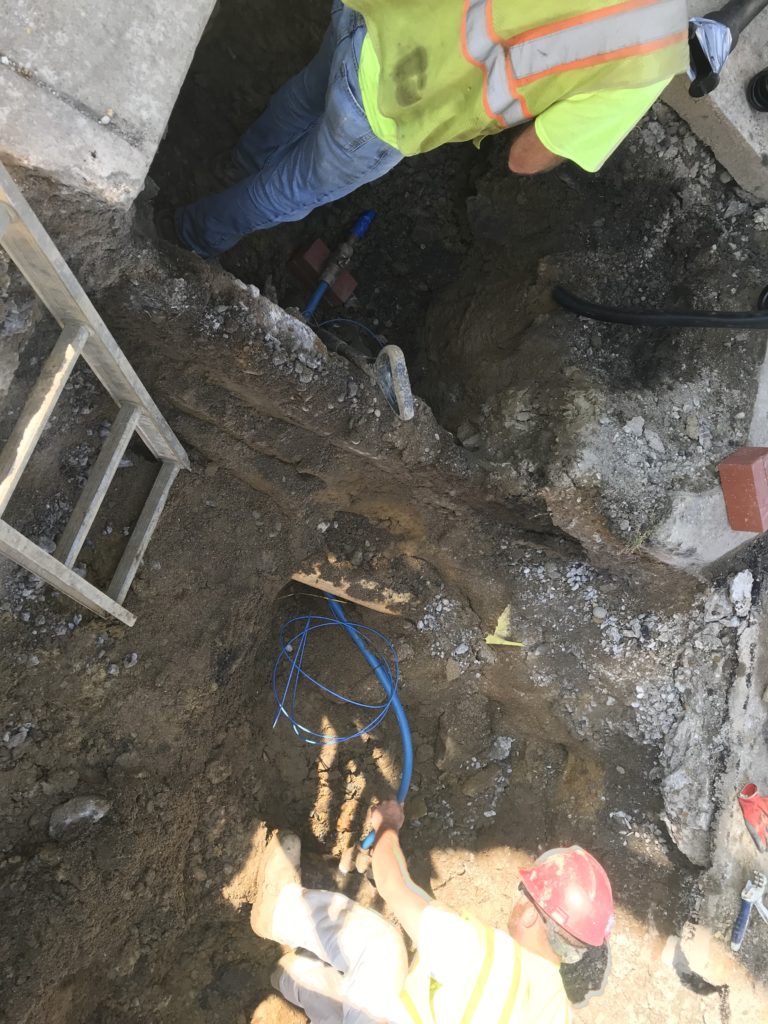
Understanding the Inventory
The Community Lead Response team at PWSA prioritizes lead line replacements during water main projects by considering both historical data about our water distribution system and public health metrics to address areas with our highest-risk residents, like children under six years and women of child-bearing age. The ultimate goal is to replace older water mains that have a high percentage of lead lines attached that are located in areas with high concentrations of at-risk populations. While population data and blood-lead level data is readily available from various government entities, comprehensive data on the locations of lead service lines is often outdated or incomplete.
PWSA has primarily relied on a collection of documents that are created when a home or business is first built. The sheet contains information on how the service line to the building was installed, including the material of the pipe. Over the years, some records may have been lost, damaged, or become outdated as the service line may have been replaced. For this reason, we had to expand our pool of data to make determinations about lead service lines.
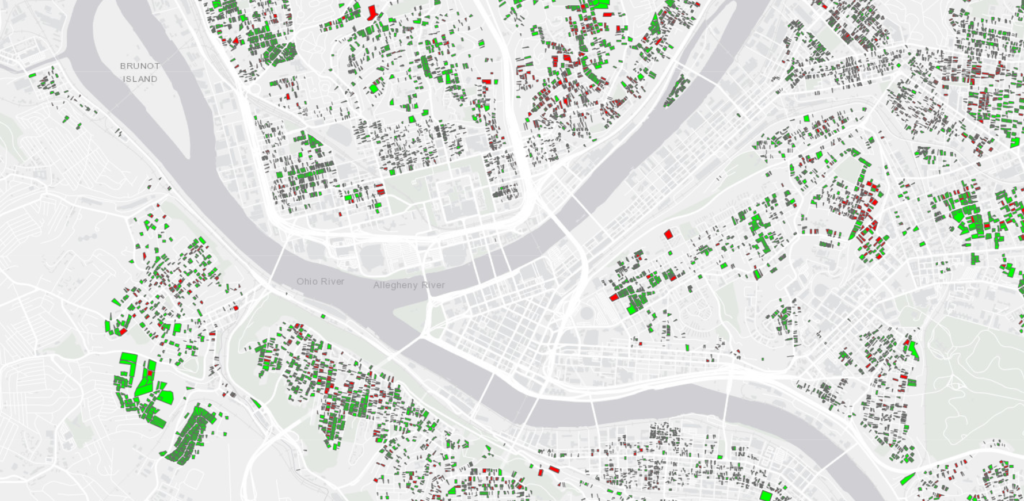
To gain a clearer understanding of where remaining lead service lines are located, we have used various historical records and investigations to find lead service lines, including:
- Curb box inspections – this is a process that involves cleaning out the curb stop, usually located in the sidewalk, to view the shut off valve attached to the service line. In some cases, this is an effective way to identify lead material.
- Meter inspection data – PWSA is replacing thousands of water meters per year. When replacing the meter, crews can view the material of the private service line coming into a building and can determine if it is lead or not.
- Property Assessment Data – the age of a building can give a better understanding if lead was used for the service line. Lead was commonly used as a pipe material in the 1920s through the 1950s.
- Water samples – a positive result of lead in a water sample taken by a resident may indicate there is a lead service line at the property.
- Historical service line documents
Comparing and organizing this amount of data is a daunting challenge and no one data point is robust enough to make accurate assessments. PWSA began this collaboration with the SCI and UCSUR to create a machine learning model that would help make sense of the various streams of information.
Determining Probability
The goal of the model is fairly simple: to provide a statistical probability of a property having a lead service line, taking into consideration all the different data points available for a given property. To do this, the research team at the University of Pittsburgh combined all available data points collected from PWSA and other demographic and historical data. They tested different predictive models to find the one that provided the most accurate predictions and “meticulously interpolated missing data, balanced the data set, and pruned weak predictors,” said University of Pittsburgh authors Saeed Hajiseyedjavadi, Dr. Michael Blackhurst, and Dr. Hassan A. Karimi.
The data is presented as a “probability” score and provides a complex patchwork of analysis distilled down to one simple metric, with each property having different inputs that combine to provide a final assessment.
Findings
After running the model and removing any data points that were not increasing accuracy, researchers found that curb box inspections and tap water lead levels were most useful in providing a strong probability of a lead service line. In other words, the various historical data points alone may or may not point to a lead line, but a recent elevated water sample and curb box inspections showing lead provide the most useful results. Additionally, geographical location, building characteristics, and available historical records were among the most useful metrics.
The Future of Lead Service Line Removal
Over the next four years, PWSA will invest over $250 million replacing aging water mains and all lead service lines attached to those old mains. To plan our replacement locations, it will be crucial to combine water main age with the findings of the machine learning model to invest ratepayer dollars wisely.
As PWSA continues to remove lead service lines, with the goal of replacing all public lead lines by 2026, it will be important to use all sources of information to target ratepayer dollars effectively. The findings of the model will help PWSA crews more effectively find lead where they dig and avoid costly excavations where lead is not found. As replacements continue and more water quality samples are collected, new data will be fed into the model to make it more effective.
“We are grateful for this partnership and the hard work of the researchers at the University of Pittsburgh,” said PWSA Chief Executive Officer Will Pickering. “This model will help us in the near term make important decisions about where we target lead service line replacements and will improve as we collect more data over time.”
- For more information on all PWSA capital improvement projects, visit https://www.pgh2o.com/projects-maintenance.
- The full machine learning model study, authored by Saeed Hajiseyedjavadi, Michael Blackhurst, and Hassan A. Karimi, is available here.
- To check the material of your service line, not including the results of the machine learning model, visit pgh2o.com/leadmap.
- To request a free lead test kit for your home, visit pgh2o.com/leadform.